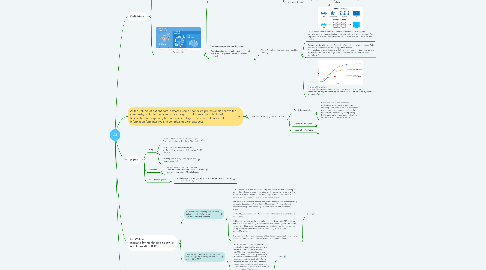
1. As the volume of patient data increases, some healthcare professionals believe the complexity of finding tools to extract insights could grow more challenging. Researchers are exploring how artificial intelligence can help draw useful information form massive and complex medical data sets.
1.1. Medical Data Mining AI Applications
1.1.1. Predictive Analytics
1.1.1.1. When companies and healthcare professionals use machine learning to analyze patient data in order to determine possible patient outcomes, such as the likelihood of a worsening or improving health condition, or chances of inheriting an illness in an individual’s family.
1.1.2. Diagnostic Analytics
1.1.2.1. Is defined by Gartner as “a form of advanced analytics which examines data or content” to determine why a health outcome happened.
1.1.3. Prescriptive Analytics:
1.1.3.1. When research firms develop machine learning algorithms to perform comprehensive analyses of patient data to improve the quality of patient management such as handling patient cases or coordinating the flow of tasks, such as ordering tests, among of medical personnel.
1.1.3.1.1. The team found a higher likelihood of IBD flares, hospitalization rates and related surgeries among individuals with low continuity of care.
2. News
2.1. NPR
2.1.1. How Can Doctors Be Sure A Self-Taught Computer Is Making The Right Diagnosis?
2.1.2. As Artificial Intelligence Moves Into Medicine, The Human Touch Could Be A Casualty
2.1.3. Scanning The Future, Radiologists See Their Jobs At Risk
2.2. Research
2.2.1. Stop Explaining Black Box Machine Learning Models for High Stakes Decisions and Use Interpretable Models Instead
2.3. AI and video games
2.3.1. Bored with your video game? Artificial intelligence could create new levels on the fly
3. Definitions
3.1. AI vs ML vs DL
3.2. Timeline
3.2.1. Machine Learning
3.2.1.1. Algorithms that analyze data, learn from it and make informed decisions based on the learned insights.
3.2.1.1.1. Machine Learning incorporates “ classical” algorithms for various kinds of tasks such as clustering, regression or classification. Machine Learning algorithms must be trained on data. The more data you provide to your algorithm, the better it gets.
3.2.2. Deep Learning — The next big Thing Deep Learning uses a multi-layered structure of algorithms called the neural network
3.2.2.1. Why is Deep Learning better than Machine Learning?
3.2.2.1.1. Doesn't need Feature Extractio. Feature Extraction is usually pretty complicated and requires detailed knowledge of the problem domain. This step must be adapted, tested and refined over several iterations for optimal results.n
3.2.2.1.2. To quote Andrew Ng, the chief scientist of China’s major search engine Baidu and one of the leaders of the Google Brain Project: “ The analogy to deep learning is that the rocket engine is the deep learning models and the fuel is the huge amounts of data we can feed to these algorithms. “
3.2.2.1.3. The Era of Big Data… The second huge advantage of Deep Learning and a key part in understanding why it’s becoming so popular is that it’s powered by massive amounts of data.
4. Dr. Waljee Institute for Healthcare Policy and Innovation (IHPI)
4.1. Association of Continuity of Care With Outcomes in US Veterans With Inflammatory Bowel Disease
4.1.1. “Continuity of care was measured using the Bice-Boxerman continuity of care index, which provides information on the dispersion of care, or the spread of a patient’s care across multiple providers, as well as care density, or the relative share of visits by each provider. The index is a scale from zero to one, with a score of zero demonstrating complete discontinuity,” says Cohen-Mekelburg. “A score of one is consistent with perfect continuity, when all visits are with the same provider.” Of the 46,665 patients with IBD identified by the team, a majority had a VA provider. “Ultimately, we narrowed our populations size down to 20,079 patients with a calculable continuity of care index within the first year of the study period, and this population was used to identify factors that were most strongly associated with low levels of continuity of care,” says Cohen-Mekelburg. The team found a higher likelihood of IBD flares, hospitalization rates and related surgeries among individuals with low continuity of care.
4.1.1.1. IHPI
4.2. Prevalence of Immunosuppressive Drug Use Among Commercially Insured US Adults, 2018-2019
4.2.1. 3% of insured U.S. adults under 65 take medications that weaken their immune systems. The analysis reveals nearly 90,000 people met the study criteria for drug-induced immunosuppression that may elevate risk for severe COVID-19 symptoms and hospitalization if they became infected. Two-thirds of them took an oral steroid at least once, and more than 40% of patients took steroids for more than 30 days in a year.
4.2.1.1. IHPI
4.3. Development and Validation of Machine Learning Models in Prediction of Remission in Patients With Moderate to Severe Crohn Disease
4.3.1. Long-term ustekinumab response may be predicted using demographic and laboratory data before week 8 of treatment, without the need for costly drug-level monitoring
4.3.2. Methods
4.3.2.1. Random forest methods were used in building 2 models for predicting Crohn disease remission,
5. Methods
5.1. Random Forests
5.1.1. Random Forests Explanation and Visualization
5.1.1.1. 5 Min vid Random Forests Explanation and Visualization